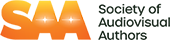
Speech by ALCS Chair and technology expert Tom Chatfield delivered at the International Federation of Reproduction Rights Organisations (IFRRO) World Congress on 3 October 2023. Chatfield discussed the ways in which debates around AI are framed, and how to think about the issues in more productive and empowering ways.
Artificial intelligence (AI) represents an extraordinary opportunity. But the way it is spoken about by some technology companies is profoundly disingenuous, as they exaggerate AI’s capabilities while minimising the words and works of humans that are fundamental to its achievements.
First, there is a common tendency in technology circles to say that things are inevitable when in fact they are just convenient – and to say things that are impossible when they are inconvenient.
A tech company will say that it is inevitable that next year AI’s will be doing this or that, when it is not inevitable at all, it is simply convenient. Or they will say that it impossible is for us to demonstrate how an AI arrives at its outputs, or to list all its training data. Yet it’s not impossible, it is just inconvenient. When we look at technology in a deterministic way, we allow tech companies to shape the process towards what suits them and their shareholders. This doesn’t need to be the case.
Second, I think it is extraordinarily dangerous when debating artificial intelligence and the world of big data to be trapped into the kind of discussions that say: “only humans are capable of doing X”, whether that is writing poetry on the human condition or telling original jokes. This is a dangerous way to defend the value of human works, words and ideas, because in terms of outputs there is virtually nothing that AI won’t be able eventually to simulate.
It is a losing battle, in other words, to rest your case on being able to say that there are certain outputs that only humans can create, as AIs are almost perfect statistical mimics. So for me, the key question isn’t what AI can do. It is what AI should do.
You can think of it like this. If there is value in literature, politics, philosophy or democratic debate, the value doesn’t just come from the end output, the final result: the value is also the process, and the involvement of human beings in that process as advocates for a particular point of view; as unique possessors of experience, worth and insight; as individuals learning and testing and improving and sharing ideas as they go.
An analogy that helps me with this is the idea of sport. Let’s say that we could develop an algorithmic model that perfectly predicts the outcome of the English Premier League, then on this basis claim football is “solved” and there’s no reason to play actual games to find out the results. Football is solved: think of all the time and money we can save but not actually having to play it! Of course, this would completely miss the point of why people watch football, play football, enjoy football, admire football, are deeply moved by it, and so on.
It is a really stupid way of thinking about football. Yet it is the way that a lot of arguments around AI in effect describe works of art, democratic processes, legal processes, civic debates, education, and so on. When we think about human values, we need to think less about outputs and more about defending the processes, stories and values that creating and debating them are bound up with.
Third, treating everything as an equal soup of undifferentiated data is not a useful way of thinking about human creations or values. There is an enormous tendency to say, well, everything is just data, your work is only a tiny part of the data, so it’s insignificant. But this is a misguided way of thinking about both human creativity and information, because not all information is created equal.
Indeed, technology itself increasingly relies upon this. ChatGPT stands for Generative Pretrained Transformer. A transformer achieves very high degrees of efficiency at pattern recognition by using what are called ‘attention’ mechanics. Attention mechanics actively allow the model to recognise that not all information, and not all elements within it, are created equal. Some patterns and pieces of information are far more important than others. And some kinds of information are actively detrimental to insight and performance.
There is an old saying in the world of computing: garbage in, garbage out. If we input things that we can’t trust, then we can’t trust the final result. Content that comes from a human mind and is of high quality, accurate and easily understood is vastly more significant than low quality content. As every human creator knows, the influence and significance of even a single work can be incalculable.
AI is in a period of transition, and in its current form it is plagued by issues such as hallucination (often-convincing outputs that actually have little or no relation to reality). AIs’ data world is insecurely related to our real world, and that is an enormous problem. To be financially viable, AI companies will need to ensure there’s a high degree of trust in their outputs. So how will they solve this problem?
People are busy working on the next generation of their models, some of which are based on identifying high quality sources and being able to display them. They aim to discriminate between verified and unverified information, and to more securely anchor the world of data to the real world.
As well as being a time of transition, then, this is a time of great opportunity for those concerned with the rights of authors. We are already seeing a convergence around principles like trust, transparency, accountability and truth. There is a growing acceptance on all sides that we need to find ways to respect creators’ intrinsic rights of recognition, consent and remuneration.
Collective Management Organisations are well placed to provide solutions to the issue of anchoring AI to the world, in terms of both inputs and outputs. If some truly ambitious collective thinking takes place now, I believe it will be perfectly possible to present a solution to many of the problems that threaten to cripple AI companies’ business models – of trust, rights, reliability and transparency – while ensuring that human creators have the right to recognition and remuneration.